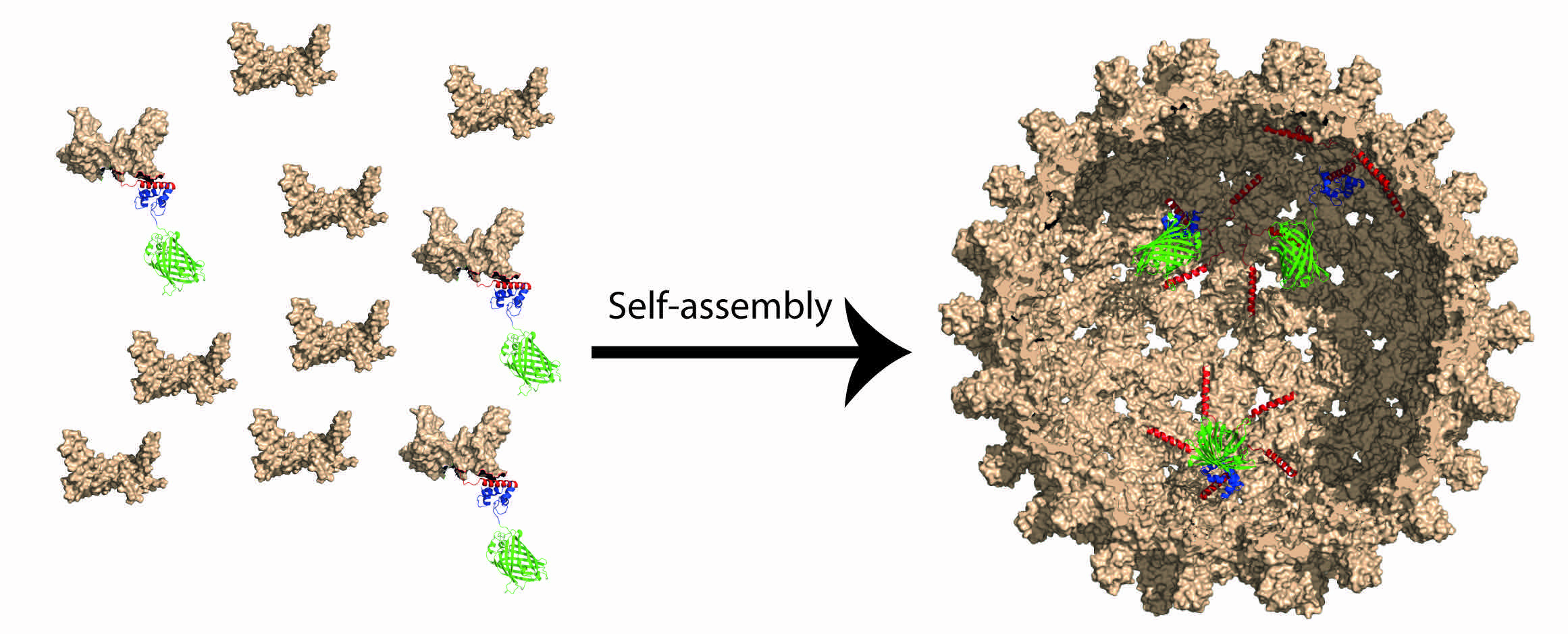
Protein design
Lizatović R, Assent M, Barendregt A, Dahlin J, Bille A, Satzinger K, Tupina D, Heck AJR, Wennmalm S, André I. A Protein-Based Encapsulation System with Calcium-Controlled Cargo Loading and Detachment. Angew Chem Int Ed Engl. 2018 Aug 27;57(35):11334-11338.
Lizatović R, Aurelius O, Stenstrom O, Drakenberg T, Akke M, Logan DT and André I. A De Novo Designed Coiled-Coil Peptide with a Reversible pH-Induced Oligomerization Switch. Structure 2016.
Norn CN and André I. Computational design of protein self-assembly. Current Opinion in Structural Biology 2016; pp 39-45.
Kaltofen S, Li C, Huang PS, Serpell LC, Barth A, André I. Computational de novo design of a self-assembling Peptide with predefined structure. Journal of molecular biology. 2015;427:550-62.
Rämisch S, Weiniger U, Martinsson J, Akke M and Andre I "Computational design of Leucine-Rich Repeat proteins with a defined geometry" Proc Natl Acad Sci, 2014, pii: 201413638.
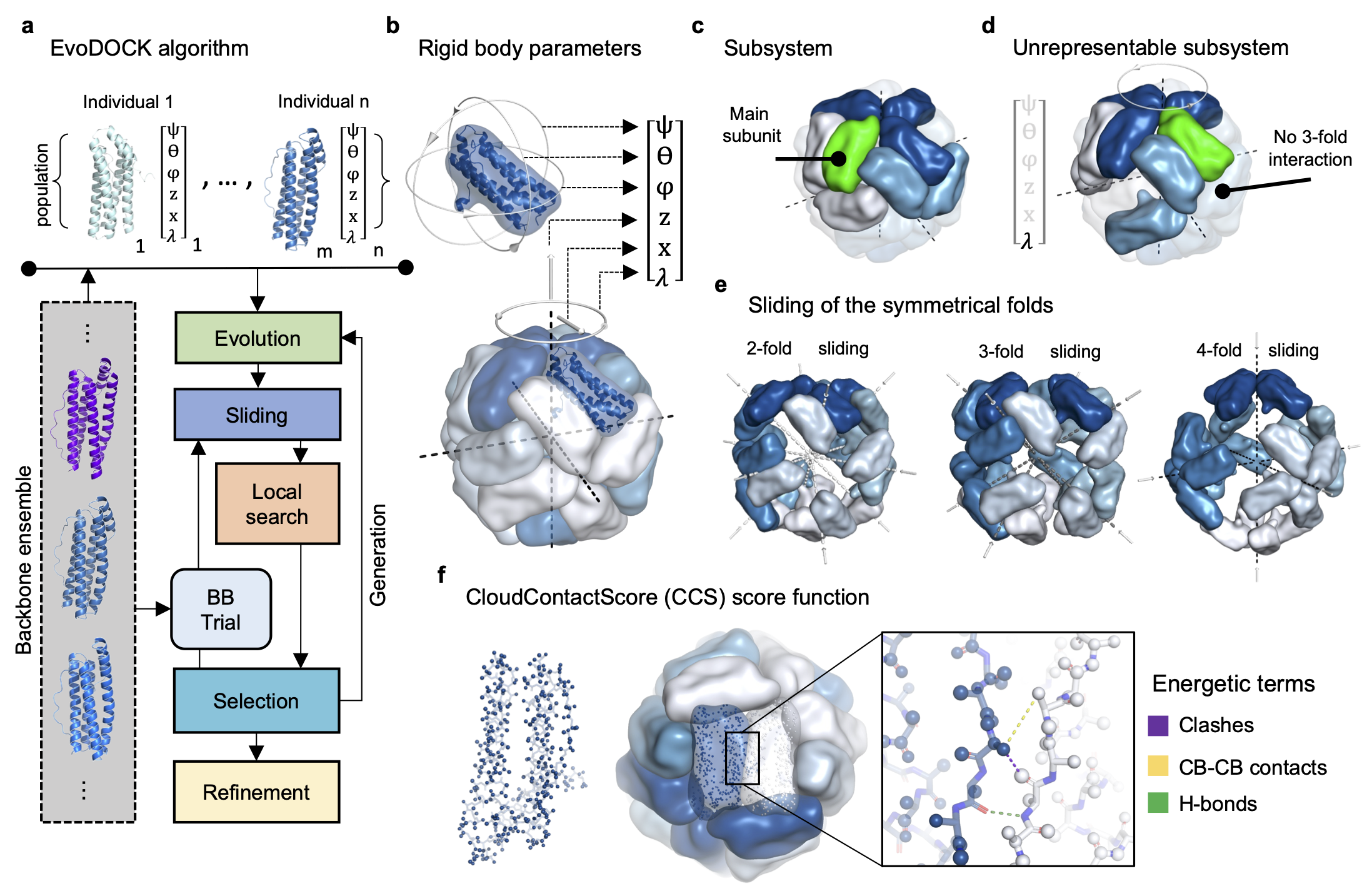
Protein Structure prediction
Protein structure prediction has been transformed by artificial intelligence methods, enabling atomistic prediction of protein structure from sequence for many systems. Nonetheless, there are still remaining challenges, such as prediction of large protein assemblies and protein complexes. We develop methods that combine machine learning with traditional docking approaches.
Jeppesen M and André I. “Accurate prediction of protein assembly structure by combining AlphaFold and symmetrical docking”. Nature Commun. 2023 Dec 13;14(1):8283.
Daniel Varela, Vera Karlin and Ingemar André. A memetic algorithm enables efficient local and global all-atom protein-protein docking with backbone and side-chain flexibility. Structure. 2022 Nov 3;30(11):1550-1558.e3.
Potrzebowski W, Trewhella J, André I. Bayesian inference of protein conformational ensembles from limited structural data. PLoS Computational Biology. 2018. 14, 12, e1006641.
André I, Bjelic S. Computational assessment of folding energy landscapes in heterodimeric coiled coils. Proteins. 2018 Apr 20. doi: 10.1002/prot.25516.
André I. Modeling the Structure of Helical Assemblies with Experimental Constraints in Rosetta. Methods Mol Biol. 2018;1764:475-489.
Potrzebowski W, André I. "Automated determination of fibrillar structures by simultaneous model building and fiber diffraction refinement". Nat Methods. 2015; doi: 10.1038/nmeth.3399
Rämisch S, Lizatović R, André I. Automated de novo phasing and model building of coiled-coil proteins. Acta Cryst D. 2015;D71.
Rämisch S, Lizatovic and Andre I. "Exploring alternate states and oligomerization preferences of coiled-coils by de novo structure modeling" Proteins, 2014. doi: 10.1002/prot.24729
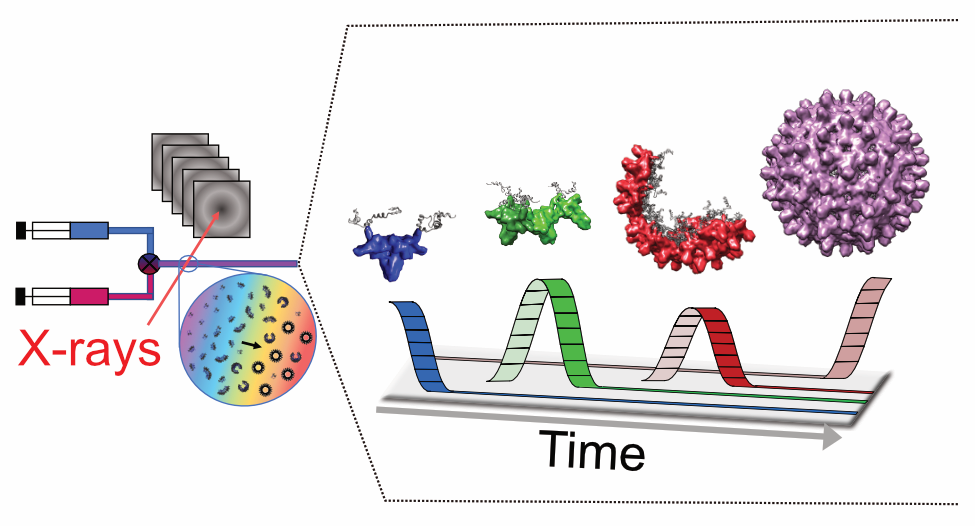
Guiding structural modeling with experimental data
Ryan C Oliver, Wojciech Potrzebowski, Seyed Morteza Najibi, Martin Nors Pedersen, Lise Arleth, Najet Mahmoudi and Ingemar André. “Assembly of Capsids from Hepatitis B Virus Core Protein Progresses through Highly Populated Intermediates in the Presence and Absence of RNA.” ACS Nano 2020 Aug 25;14(8):10226-10238.
Potrzebowski W, Trewhella J, André I. Bayesian inference of protein conformational ensembles from limited structural data. PLoS Computational Biology. 2018. 14, 12, e1006641.
André I. Modeling the Structure of Helical Assemblies with Experimental Constraints in Rosetta. Methods Mol Biol. 2018;1764:475-489.
Boelt SG, Norn C, Rasmussen MI, André I, Čiplys E, Slibinskas R, Houen G, Højrup P. Mapping the Ca(2+) induced structural change in calreticulin. J Proteomics. 2016 Jun 16;142:138-48.
Potrzebowski W, André I. "Automated determination of fibrillar structures by simultaneous model building and fiber diffraction refinement". Nat Methods. 2015; doi: 10.1038/nmeth.3399
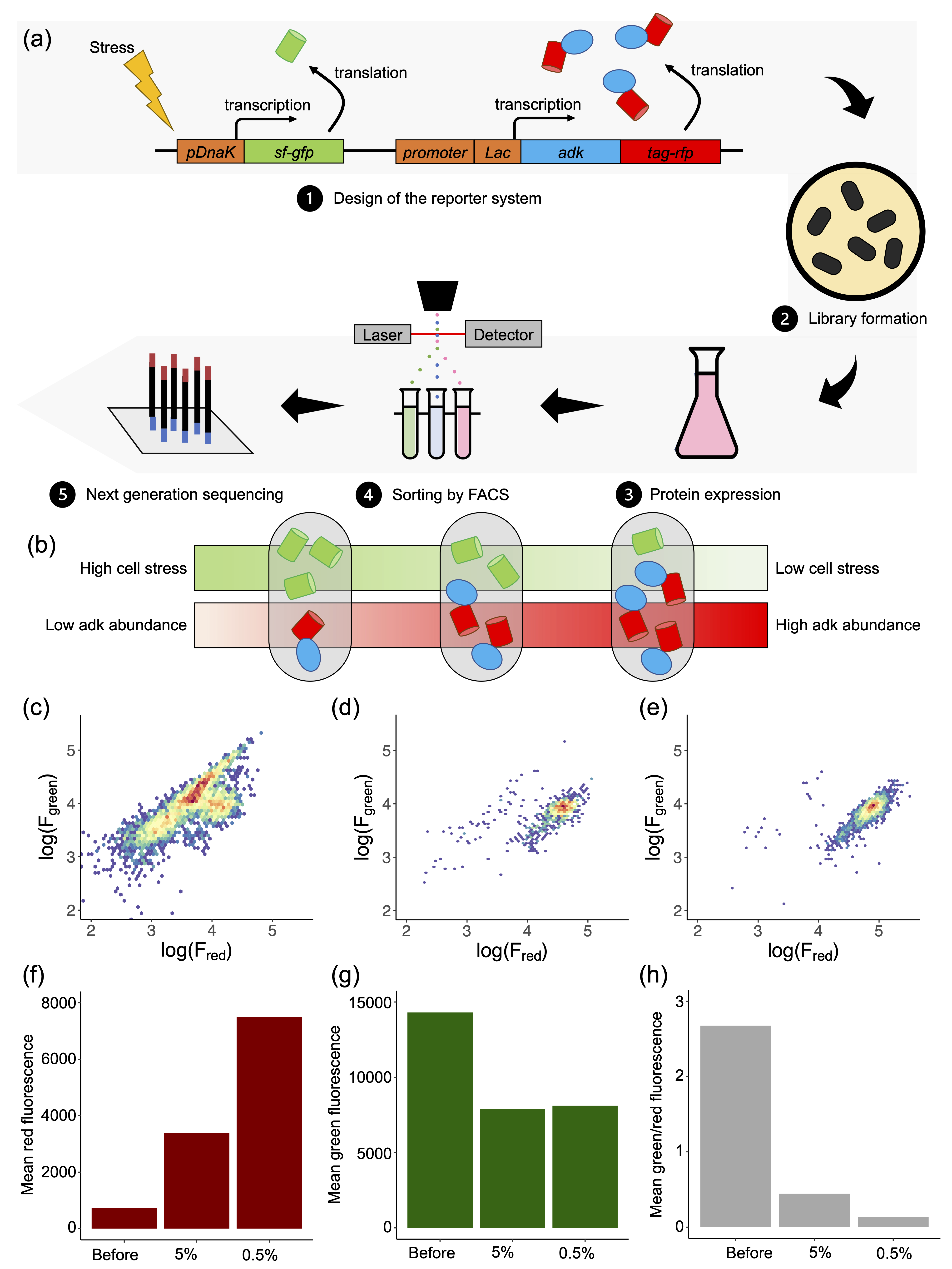
High-throughput protein characterization
We are interested in characterizing the relationship between sequence - codon and protein - and biophysical properties at large scale. We develop methods for high-throughput screening of protein sequences using DNA libraries and fluorescencence assays.
S Christensen, C Wernersson, I André. “Facile Method for High-throughput Identification of Stabilizing Mutations“. Journal of Molecular Biology 435 (18), 168209.
Signe Christensen, Sebastian Rämisch and Ingemar André. “DnaK response to expression of protein mutants is dependent on translation rate and stability.” Communication Biology (2022), 5 (1), 1-13.
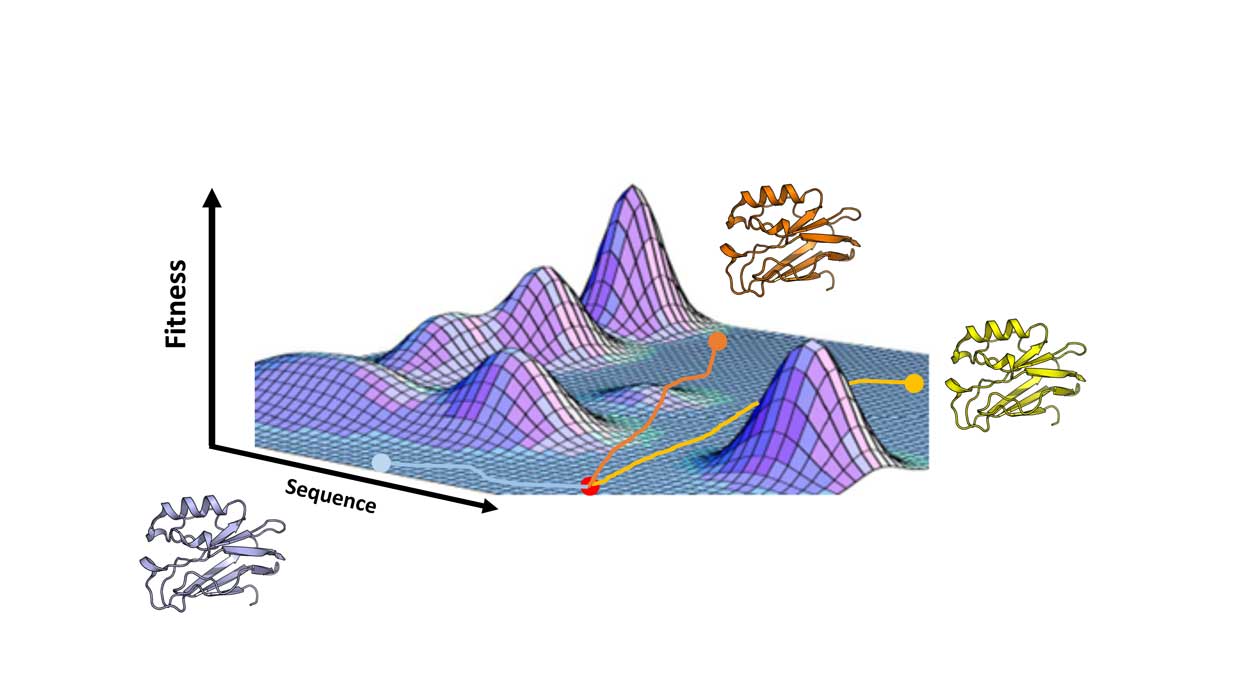
Evolution
Norn C and André I. Atomistic simulation of protein evolution reveals sequence covariation and time-dependent fluctuations of site-specific substitution rates. PloS Comput Biol. 2023 Mar 24;19(3):e1010262.
Christoffer Norn, Ingemar André Douglas L. Theobald. A thermodynamic model of protein structure evolution explains empirical amino acid substitution matrices. Protein Science. 2021 Jul 4. doi: 10.1002/pro.4155.
André I, Strauss CEM, Kaplan DB, Bradley P, and Baker D. "Emergence of symmetry in homooligomeric biological assemblies." PNAS 2008 105 (42) 16148-16152.